Data analytics is a fundamental aspect of data science that revolutionizes healthcare by providing valuable insights into patient health, addressing various healthcare data challenges. By leveraging data science in healthcare, industry professionals can improve the correctness of diagnoses and provide more personalized treatment plans.
Unfortunately, not all healthcare departments utilize healthcare data science and modern analytics techniques to their full extent. Due to the social stigma, mental healthcare has remained on the sidelines of industry modernization for many years. Even though society is now more interested in mental healthcare, many people cannot afford professional help and experience mental health issues.
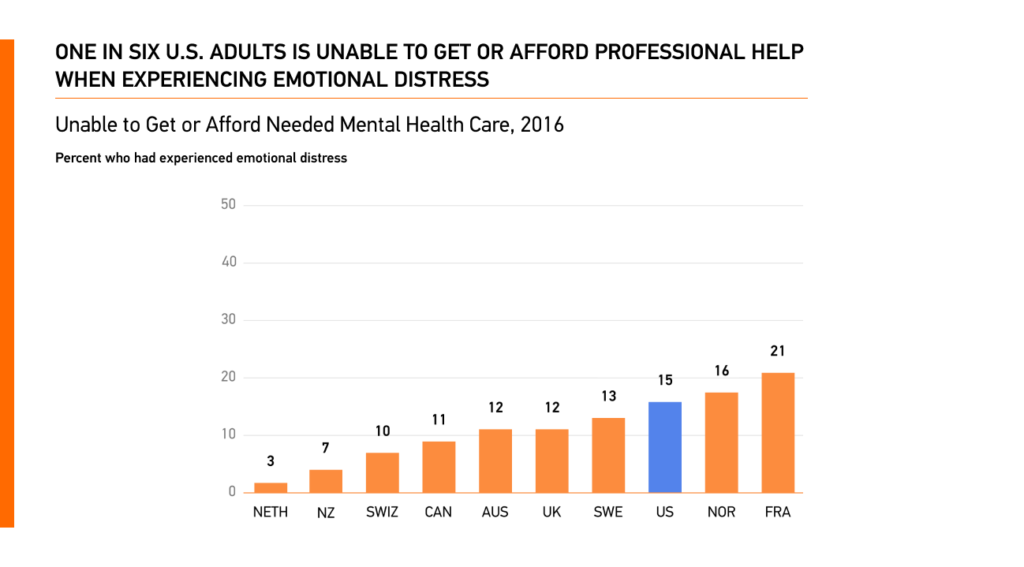
In this article, we will review a practical use of data science that can improve mental healthcare and ensure the effective use of patient data by using cost-effective data analytics techniques.
DATA SCIENCE IN HEALTHCARE
Healthcare data science uses artificial intelligence, machine learning, and data analytics techniques to draw insights from large amounts of data from various sources. It involves collecting, analyzing, and interpreting healthcare data to support clinical decision-making, research, and public health initiatives.
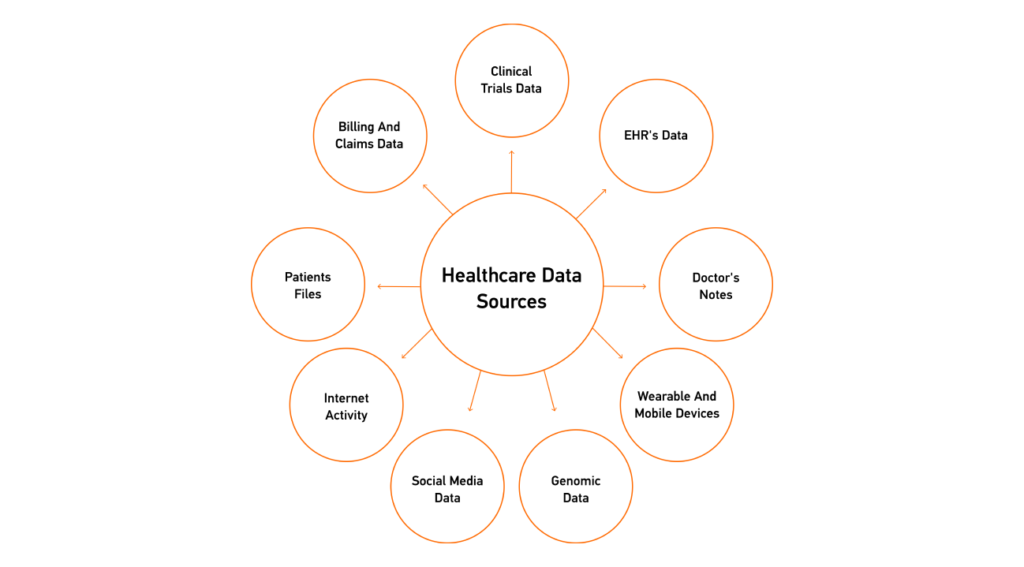
How can the main healthcare data sources improve healthcare?
- Clinical trial data: allows for testing the efficacy and safety of new treatments against a control group. Data science techniques can help researchers identify patterns and associations in clinical trial data to develop more effective treatments.
- Patient files, doctor’s notes, EHR data: Electronic Health Records (EHRs) contain vast patient data, including medical histories, diagnoses, treatments, and lab test results. Data science can help doctors better understand a patient’s condition.
- Billing and claims data: Insurance claims data includes information about patient demographics, diagnoses, and treatments. Insurers can use identified patterns and trends to improve risk assessment and pricing by analyzing this data with data science methods.
- Genomic data: DNA sequencing data includes information about genetic predispositions to diseases. With the help of data science, researchers can identify genetic markers for specific conditions to develop more targeted treatments.
- Wearable and mobile devices: Wearable devices such as smartwatches and fitness trackers can track various health metrics, including heart rate, activity levels, and sleep patterns. This data can be used for behavioral health data analytics to extract insights from this data to develop personalized patient care plans.
- Social media data and internet activity: Data science techniques (e.g., machine learning and natural language processing) can be used to analyze social media and internet activity to identify undiagnosed mental illnesses or track disease outbreaks.
Does mental healthcare lag behind?
Despite the promising opportunities AI brings to mental health care and health data analytics, mental healthcare still struggles to get the necessary attention from society. By delaying the modernization of mental healthcare, society worsens the economic performance of many countries because mental illness affects millions worldwide and significantly impacts the economy.
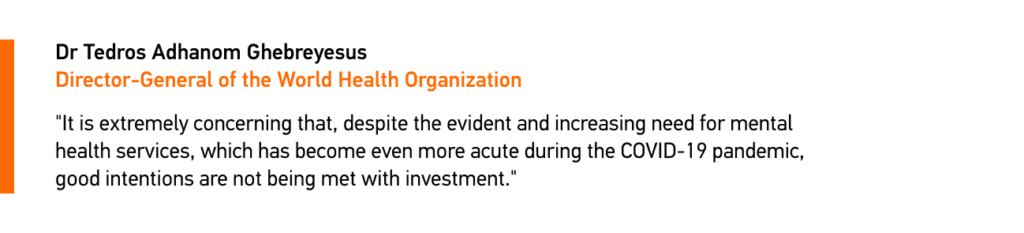
Globally, depression and anxiety lead to a loss of nearly 12 billion workdays, causing an estimated cost of $1 trillion in productivity losses.
The World Health Organization (WHO) set new targets for 2030 to improve mental healthcare, highlighting the urgent need for mental health to be prioritized and integrated into all healthcare and emergency planning aspects.
We couldn’t be more thrilled because the additional attention by WHO can initiate an investment in mental healthcare and encourage applying data science techniques to mental healthcare data to reduce the economic burden of mental illness.
WHY DO MENTAL HEALTH AND DATA SCIENCE MAKE THE PERFECT MATCH?
Mental healthcare is severely misunderstood
Regarding healthcare, data science is particularly effective in tackling misunderstood areas because of its ability to process and analyze large amounts of complex data to identify patterns and relationships that may not be apparent to humans. Mental healthcare is an area with still much to learn, making it a perfect match for data science.
Despite the prevalence of mental health disorders such as depression and anxiety, there are still significant gaps in our understanding of how they develop and what treatments best support those affected. Using data science techniques will help uncover the information traditional methods may have missed. Mental healthcare is an area that specifically requires behavioral data analytics.
Behavioral health data analytics involves collecting and analyzing user data to gain insights into how they interact with software (e.g., how the user moves their mouse), using improved analytics capabilities in artificial intelligence. Applying data science techniques such as machine learning in healthcare and predictive modeling to patient behavioral data can help identify red flags for mental disease and suggest help for people in the early stages.
However, one of the key challenges in behavioral health data analytics is ensuring the ethical and responsible use of sensitive user data, as well as maintaining patient privacy and data security throughout the process.
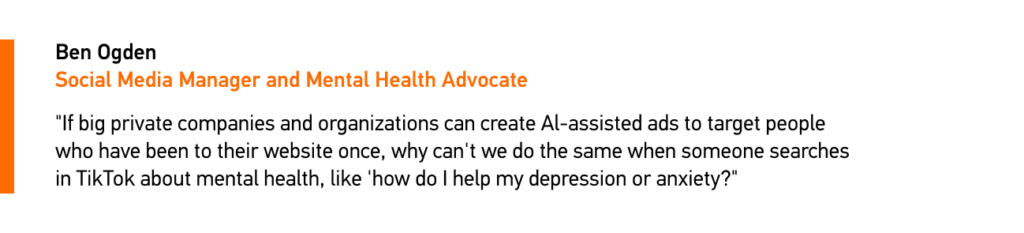
Wearables and internet activity data provide up-to-date information on physical activity, sleep, heart rate, interests, social interactions, and mental state. Analyzing this data can help researchers identify correlations between behaviors and mental health outcomes, leading to new insights and treatment approaches.
A massive gap between the onset and first treatment
The Health Services Research shows that the median number of years between the onset of a mental disorder and first treatment contact is 11 years. Hence, many aspects of healthcare data that the human eye cannot catch lead to such large gaps.
Unsupervised machine learning algorithms identify data patterns and relationships without prior knowledge or supervision. Applying data analytics techniques, such as clustering and anomaly detection, is perfect for understanding mental health conditions. Moreover, unsupervised machine learning is a cost-effective solution compared to other methods, which is essential due to the low funding for mental healthcare.
However, the value of data privacy in healthcare and ethical considerations should always be the focus when developing and refining data processing methods. The responsible use of healthcare data is crucial for closing the mental health diagnosis and treatment gaps and improving outcomes.
USE CASE: DATA SCIENCE FOR IMPROVED DIAGNOSIS AND TREATMENT OF PTSD IN UKRAINE
In the WHO’s report on the Ukraine crisis, the organization shared the potential effectiveness of using mental health and psychosocial support services (MHPSS) for people with high levels of PTSD, depression, and anxiety. However, the level of emotional distress and other mental health issues is extremely high due to the Russian invasion.
Moreover, Ukrainians with PTSD and other mental health conditions face significant barriers to care, which is unsurprising. Planning a doctor’s appointment is challenging since Ukrainians have had to use military shelters nearly every day for over a year. Moreover, Ukraine requires cost-effective methods for mental disease diagnosis so that they don’t overload the war-affected economy.
Unfortunately, covering all mental problems seems unrealistic due to the tremendous amount of Ukrainians needing help. However, the clever use of data science can help identify individuals requiring urgent mental health intervention, resulting in the more effective use of MHPSS.
The use of machine learning algorithms was applied for analyzing words and emojis to identify people in immediate need of help
The Crisis Text Line (CTL) released a study on how they have implemented machine learning algorithms to analyze words and emojis used by texters to identify individuals needing help. They analyzed a dataset of over 129 million text messages between texters and counselors on the CTL platform.
According to the CTL’s findings, the likelihood of being in a life-threatening situation was 4.4 times higher for the pill emoji (💊) than for the word “suicide.” Similarly, the intensely crying emoji face (😭) is also considered high-risk.
How can this help with PTSD in Ukraine?
Implementing machine learning algorithms to analyze words and emojis could also benefit Ukraine and help those with PTSD at risk of worsening their mental health condition. In addition, this method removes a load from healthcare professionals by automating the analysis of data, which reduces costs and improves the speed of the diagnosis process.
What can obstruct the advancement of behavioral health data analytics?
Unfortunately, there are several significant obstacles for applying health data analytics techniques to mental health data. Stigma and data privacy concerns are the most problematic aspects affecting the timely detection of mental conditions.
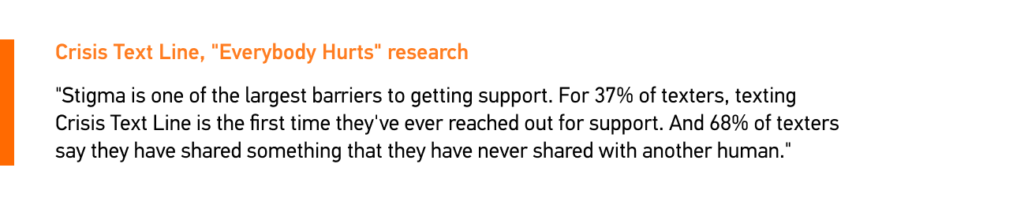
The desire to keep mental healthcare data as confidential as possible also does not help the situation. The possibility of mental healthcare data leaks frightens people so much that they are generally reluctant to turn to healthcare professionals for help.
Luckily, our team knows exactly how to overcome these barriers and unleash the potential of health data analytics.
OVERCOMING THE TWO MAJOR DATA ANALYTICS CHALLENGES IN MENTAL HEALTHCARE
1. Stigma
Patients may hesitate to share their mental health information due to fear of discrimination or negative consequences in case of data exposure. Anonymizing patient data will make patients feel more secure in sharing their data, knowing it will protect their identity.
Further analytics of the collected anonymized data will help define relations between symptoms, age, wearables data, internet activity, and other data that healthcare professionals cannot handle independently. But, most importantly, it encourages people to share their data for society’s good without risking exposing sensitive health information.
In the National E-health System, Edenlab’s experts used a pseudonymization mechanism recommended by the European GDPR regulation to separate personal and medical data. This approach ensures that even if a potential intruder gains access to medical data, it would be impossible to link it with a specific patient.
2. Data privacy
Another challenge in mental health data processing and sharing is regulatory compliance in healthcare. For example, the Health Insurance Portability and Accountability Act (HIPAA) and the Office of the National Coordinator for Health Information Technology (ONC) regulations set strict data privacy rules and requirements for using EHRs in a way that is not at odds with patients’ data security requirements.
The Kodjin FHIR Server is designed to comply with both HIPAA and ONC regulations. The server works as a safe and scalable repository of sensitive healthcare data that can securely handle large volumes of health information in a way that complies with major healthcare data privacy laws.
Edenlab’s solutions provide a way to effectively collect, process, and share sensitive patient data while maintaining privacy and security. Contact us to unlock the power of healthcare data with national-level data management solutions today.