Artificial intelligence in healthcare has been changing the industry recently. This is well illustrated by the global mental health app market size, expected to grow from $6.2 billion in 2023 to $17.5 billion in 2023 at a compound annual growth rate (CAGR) of 15.9%. The global economic losses caused by mental health issues have affected this impetus in developing mental health apps.
The World Health Organization (WHO) reports that every year, depression and anxiety result in the loss of approximately 12 billion working days globally, which costs around $1 trillion in lost productivity.
In addition, the COVID-19 outbreak and the eventual global lockdown showed the need to search for new ways to treat patients without a brick-and-mortar hospital. The accessibility and efficiency of at-home hospitals have acquired new meaning since experts report that isolation worsens an individual’s mental health conditions.
Thankfully, developers of mental health apps and society have actively tried to cope with this problem. According to a report from the Okta webinar “Businesses at Work 2023” apps like LiveWell from Optum and Modern Health, which have been experiencing the fastest growth in customer base, are up 32% and 30% year-over-year, respectively.
Moreover, Apple is developing an app for mental and physical health that allows users to track their daily activities. This application will provide journaling suggestions and other personalization options. Apple aims to use AI and technology to promote well-being and provide personalized support, contributing to the transformation of mental health care.
When power players like Apple invest in developing AI-based mental health applications, you can tell the game is worth the candle. In this article, we share three examples of using AI in the medical field, talk about the role of AI in healthcare, define the relationship between data interoperability and artificial intelligence in hospitals, and demonstrate the potential of using AI for the following mental health conditions:
- Alzheimer’s disease
- Major depressive disorder
- Post-traumatic stress disorder.
Understanding these applications is crucial for achieving ISiK Compliance, as it ensures that AI implementations align with standardized data exchange protocols in healthcare systems.
Unfortunately, many mental health conditions are still missing the understanding of the root causes of pathological changes and ways to reverse these changes. However, the early diagnosis of many psychiatric disorders gives patients and their caregivers precious time to adjust while the patient can still actively engage in the treatment process.
AI FOR ALZHEIMER’S DISEASE: AN INEXPENSIVE, SCALABLE, AND NON-INVASIVE INSTRUMENT FOR EARLY DETECTION
Why is early detection important for Alzheimer’s disease treatment?
The global increase in age longevity has resulted in the escalation of Alzheimer’s disease, known for its destructive impact on older adults’ memory, thinking, and behavior. According to the WHO, Alzheimer’s disease is the most common form of dementia (contributes to 70% of cases). Apart from affecting people with Alzheimer’s, the disease lowers the quality of life for family members.
A study of the identifying risk factors for depression among Alzheimer’s caregivers showed that in the early stages of the disease, first-degree relatives as caregivers did not demonstrate a higher rate of depression than controls. However, as the disease advances, the rate of depressive symptoms in spouses rose to 30% compared to controls, who report only a 1% rate of depression.
Another research on early intervention in Alzheimer’s disease showed the benefit of early diagnosis from a business perspective. When diagnosed between three and nine years before the standard intervention, the net economic benefits of earlier intervention increase by around 17% per year.
Thus, early diagnosis of Alzheimer’s can save a lot of healthcare dollars and improve the quality of life for people with the condition by providing them with necessary medications and procedures. Moreover, by detecting Alzheimer’s in its early stages, we can reduce depression among caregivers and family members so that they can care better for themselves and their loved ones with Alzheimer’s.
The first deep learning model to detect Alzheimer’s disease from retinal photographs at early stages.
The overview
A recent study illustrates the potential of AI in health and medicine, specifically for Alzheimer’s disease detection, promising to become a non-invasive instrument for detection, which can lower the cost of the diagnosis process and boost its scalability. Dr. Carol Y. Cheung, a neurologist and researcher at the Moorfields Eye Hospital in London, led the research.
Researchers have been studying the relationship between Alzheimer’s disease and the retina as their main focus for many years. Thanks to the extensive experience in researching Alzheimer’s from the ophthalmology perspective, the research resulted in the first deep-learning model capable of detecting Alzheimer’s disease from retinal photographs.
Methods
- A total of 12,949 retinal photographs were collected from 648 patients with Alzheimer’s disease and 3,240 healthy controls.
- The collected photographs were used to train, validate, and test the deep learning model in detecting subtle changes in the retina associated with the development of Alzheimer’s disease.
- Researchers evaluated the algorithm by using an internal validation dataset and testing datasets.
- The researchers conducted subgroup analyses to evaluate how eye disease and diabetes affected the algorithm’s performance.
Result
- In the internal validation dataset, the deep learning model demonstrated an accuracy of 83.6%, sensitivity of 93.2%, and specificity of 82%.
- The model could differentiate between participants who were amyloid β positive and those who were amyloid β negative with accuracies ranging from 80.6% to 89.3%.
- In subgroup analyses, the discriminative performance of the model was improved in patients with eye disease compared to those without (accuracy of 89.6% versus 71.7%) and in patients with diabetes compared to those without the disease (accuracy of 81.9% versus 72.4%).
This study demonstrates the potential of AI in medicine, particularly in Alzheimer’s disease diagnosis, by utilizing deep learning algorithms trained on retinal photographs. Hence, the sooner researchers validate these findings, the sooner healthcare professionals can apply these technologies to global mental health improvement.
AI AND MEDICINE: USING MACHINE LEARNING IN HEALTHCARE FOR DETECTING SIGNS OF DEPRESSION AT EARLY STAGES
Is depression dangerous, and does it requires early intervention?
Major depressive disorder (MDD), or depression, is another primary concern of the World Health Organization. The WHO’s comprehensive mental health action plan 2013-2030 reports that MDD affects more than 300 million people worldwide. Depression accounts for over 4% of the global disease burden and is among the most prevalent psychiatric disorders globally.
The American Journal of Managed Care reported the benefits of early and appropriate treatment for depression. Particularly, several studies showed that early diagnosis of depression in primary care settings can improve social function, increase productivity, and reduce workplace absenteeism.
Unfortunately, despite the significant burden of depression, many patients do not receive adequate treatment to achieve remission. Therefore, practical strategies for achieving remission should comprise various procedures, which might include AI-based tools soon.
This AI-backed startup can detect signs of depression by listening to short clips of a speech.
The overview
- The researchers used machine learning algorithms to evaluate voice responses to the “How was your day?” question and self-reported PHQ-9 scores. PHQ-9 is a patient-administered variation of the PRIME-MD diagnostic tool for prevalent mental illnesses. For example, PHQ-9 evaluates each of the nine DSM-IV criteria for depression and assigns a score ranging from “0” (not at all) to “3” (nearly every day). One recent research study in psychiatric hospitals in China showed the reliability of the PHQ-9 for assessing the severity of depression.
- Depression-related care accounts for 10% of primary care encounters, with general practitioners correctly identifying patients for screening in only 47.3% of cases.
- The study recruited individuals over 18 years old in the United States and Canada via social media to develop a machine-learning model.
- The study aimed to develop a model that could detect signs of depression in individuals who self-report severe depression, using voice responses to the prompt “How was your day?” and the PHQ-9 instrument (one recent research study in psychiatric hospitals in China showed the reliability of the PHQ-9 for patients with major depressive disorder).
Methods
- The training and validation analysis included individuals with PHQ-9 scores greater than 20.
- Researchers trained a logistic regression model with TF-IDF features on 1,202 EMR chat messages, among which 382 were categorized as potential crises, including suicidal or homicidal ideation, domestic violence, or non-suicidal self-injury.
- The model was trained iteratively with the training data before validation, where prediction outputs were scaled between 0 and 1.
Results
- A trained AI mechanism successfully detected signs of severe depression from <45 seconds of speech.
- Further evaluation was recommended for a group of 73 participants.
This research highlights the potential for AI in healthcare to detect depression, particularly in virtual care settings. The results reveal the feasibility of employing AI-based techniques, specifically machine learning, in identifying voice biomarkers for depression. Thus, AI can help to prioritize and detect high-risk patients with severe depression through telehealth channels for further screening.
AI FOR PTSD: A DIAGNOSTIC TOOL FOR EARLY DETECTION OF PTSD
It is hard to believe that during the active implementation of AI technology in healthcare, international newspapers headlined Europe’s largest unprovoked invasion since World War II. Yet, the world must react quickly to properly detect and treat mental health issues among soldiers and civilians in Ukraine.
According to The Economist, approximately 100 soldiers seek treatment every week for symptoms related to battlefield trauma. These symptoms include sleeplessness, nightmares, flashbacks, and intense guilt for surviving when many of their comrades did not.
Even though PTSD appears to be the least tragic consequence of the Russian invasion of Ukrainian lands, the need to develop efficient and accessible tools for early diagnosis of PTSD is undeniable. According to the WHO World Mental Health Surveys, recovering from PTSD is notably sluggish in war. Therefore, an artificial intelligence solution may become an accessible and cost-effective instrument for diagnosing and treating the disease.
Accessing the efficiency of an AI-based diagnostic tool in detecting symptoms of mental health disorders in Ukrainian citizens impacted by the ongoing conflict.
The overview
- The study identified symptoms of mental health disorders among Ukrainian citizens affected by the ongoing invasion by Russia.
- The trial evaluates the effectiveness of an AI-powered diagnostic tool in detecting PTSD symptoms at early stages and its potential to replace current measures in the future.
- The platform provides tele-visit and e-consent features.
Methods
- The study employed adaptive design and biometric onboarding technology to optimize remote participants’ pre-screening and consenting process.
- Researchers used quota sampling to collect a sample of 10,000 Ukrainian citizens, including those impacted by the war, to ensure representativeness.
- The platform captured unstructured data, such as voice and video recordings, as well as traditional data points via its EDC and EPRO modules.
Results
- over 10% of the participants confirmed PTSD following structured clinical interviews with practitioners.
- Incorporating an AI-screening panel into self-assessment tools resulted in over 25% improvement in sensitivity.
- The use of AI in healthcare for PTSD diagnosis also enhanced the specificity by over 60%.
Improving the sensitivity and specificity of existing measures can improve the early detection of PTSD symptoms. AI-powered diagnostic tools incorporate unstructured data such as voice and video recordings to capture a more comprehensive picture of an individual’s mental health. In addition, the remote and virtual conduct of clinical trials can boost patient participation, reduce trial costs, and assist the development of new treatments.
DATA INTEROPERABILITY IS THE KEY TO THE SUCCESSFUL USE OF AI-BASED TOOLS
During the HIMSS23 discussion, Tina Manoharan, VP of Data & AI Center of Excellence with Philips, discussed the symbiotic relationship between artificial intelligence and healthcare data interoperability and the use of AI for healthcare.
How is AI used in healthcare?
In healthcare, artificial intelligence is programmed to execute numerous procedures on healthcare data. Hence, AI requires access to various real-world data sources to generate meaningful insights from data, making interoperability a critical factor. By integrating interoperable AI solutions into existing workflows, establishments can use these tools to their fullest potential and provide continuous feedback for improvement.
Also Read: Role of Virtual Reality in Healthcare
To effectively address the challenges of artificial intelligence in healthcare, AI requires guidance and validation of its results. The collaboration between AI technologies and the FHIR validator, developed from scratch with the Kodjin FHIR Server, forms a valuable partnership, ensuring accurate outcomes and efficient performance.
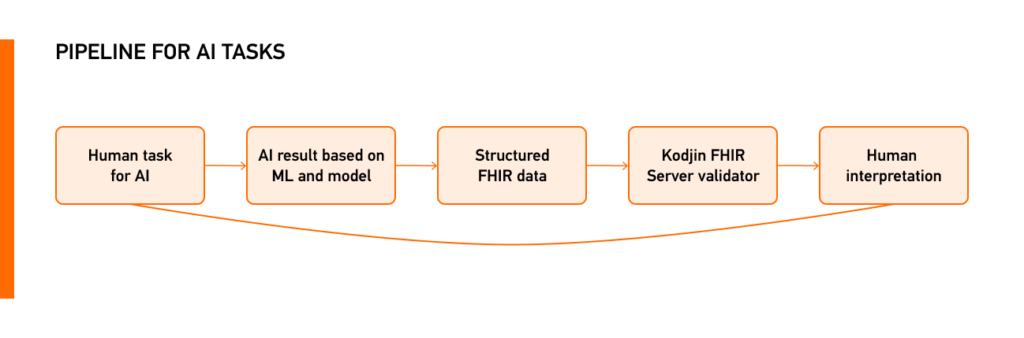
Like AI and healthcare data interoperability, the symbiotic relationship between Edenlab and Neurons Labs ensures the development of interoperable AI solutions in healthcare. Creators of the FHIR-based national-level healthcare data management tools at Edenlab and the AI/ML experts at Neurons Labs are ready to utilize their extensive experience in data interoperability, artificial intelligence, and machine learning to turn your AI startup into an enterprise-level project for transforming healthcare through artificial intelligence.